Making use of artificial intelligence takes more than just buying the technology and flipping the “on” switch. Companies need to understand the goals they want to accomplish — and ensure that they have the right data to get there.
“You can’t expect the AI to come up with the solution for you,” said Phil Crawford, Chief Technology Officer at Nashville-based CKE Restaurants. “You really have to think about your end goal. Are you trying to achieve speed? Accuracy? A frictionless environment?”
CKE operates thousands of Carl’s Jr. and Hardee’s restaurants around the world and wanted to use artificial intelligence to help with drive-through automation. That goal required the aggregation of different kinds of data from different sources, including drive-through timers, personnel information, sales data, and audio from the drive-through speakers.
As a result, the first step the company took was to create a data lake to aggregate the data sources. CKE opted for Snowflake as their platform and began implementing it in the last quarter of 2021.
“We couldn’t skip it,” said Crawford. “There’s no other way to do it. You have to have that data layer in there.”
If the company relied purely on the data in a single database, the resulting solution would have been much more limited.
“I could put the AI engine in the point of sale,” said Crawford. “But it wouldn’t do any good. You need all the components together.”
The AI functionality was running in the second quarter of this year, he said. “I can’t share the exact results, but it’s been positive. We’re benchmarking against our internal KPIs and we’re pleased with the response.”
CKE isn’t alone in discovering that AI success requires a new approach to managing data. In the past, enterprises have optimized their data around the needs of traditional structured databases. Today’s AI systems, however, require more data, different kinds of data, and need that data sooner than ever before.
Challenges of the traditional data environment
Traditional databases typically require rigid data pipelines that carry data from originating systems and classify and massage it to fit into neatly structured data schemas. But AI models often need unstructured data such as video, audio, images, raw scientific data, social media, documents, and unstructured logs, said Andy Thurai, vice president and principal analyst at Constellation Research.
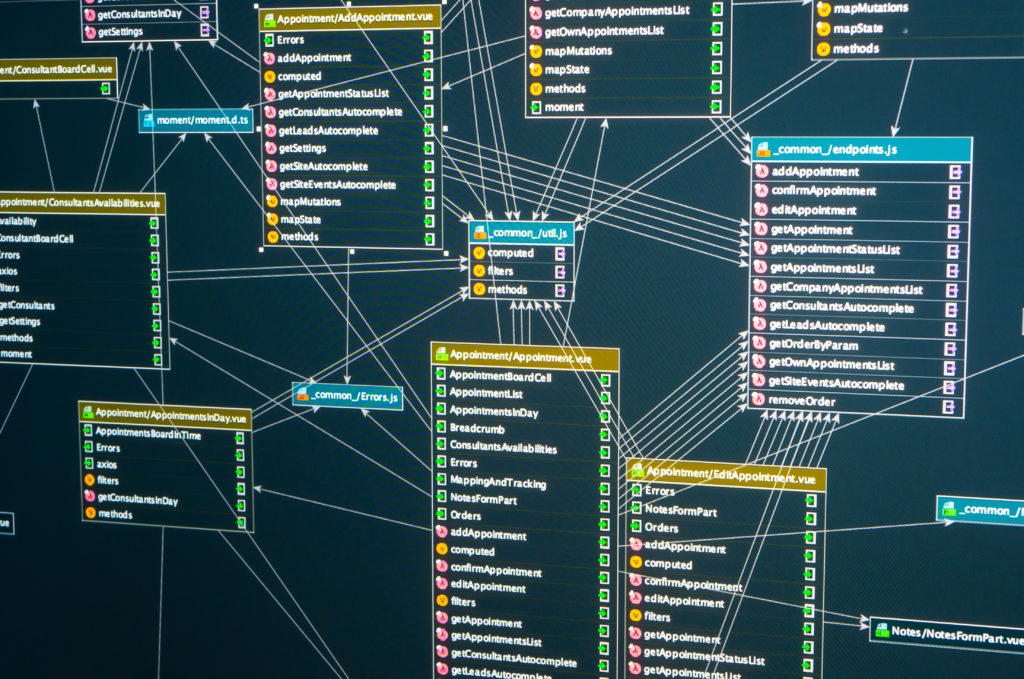
“Unstructured data can’t fit into predefined data models,” he said. “And unstructured data can’t be queried using SQL and other relational methods.”
Plus, video and audio files and other types of unstructured data can take up a great deal of space, he said.
“Cost is a major factor,” he said. “Given the massive size of storage requirements, the storage needs to be much cheaper than traditional systems.”
Finally, traditional databases require a great deal of preparatory work. Data elements must be of particular data types and have particular relationships to other data elements. This takes time to set up. As a result, the traditional environments may be a poor fit for AI environments where data scientists need to access data and build models quickly.
“You can’t do that if you’re building pipelines to batch access, move and combine data,” said Forrester analyst Michele Goetz. “And data scientists are looking for new relationships. They don’t want the data over-prepared. They want the opportunity to model the data for their own purposes.”
Another challenge of the traditional data environment is that access is limited to specific business applications.
“With AI, there’s a lot more democratization in how you prepare and model data,” said Goetz. “It’s not just constrained to the data management organization. You have analysts, data stewards, governance teams, citizen scientists.”
How to put AI first
An AI-first data strategy is going to incorporate structured, semi-structured, and unstructured data, said Bradley Shimmin, chief analyst for AI platforms, analytics, and data management at Omdia.
“Companies that are prioritizing AI outcomes need to architect a data architecture that doesn’t care what that format is of the data and where it’s coming from,” he said.
“Companies that are prioritizing AI outcomes need to architect a data architecture that doesn’t care what that format is of the data and where it’s coming from.”
Bradley Shimmin, Chief Analyst for AI platforms, Analytics, and Data Management at Omdia
That data structure might be a data lake or lakehouse, or a data fabric. A data lake collects all the raw data before it’s processed into the rigid formats of the relational database. It’s often a lower-cost type of storage, as well, allowing companies to collect more data. A lakehouse combines the best features of a data lake and a data warehouse, supporting both unstructured data and structured databases. A data fabric allows a company to access the data wherever it might reside, using APIs — application programming interfaces — to move the data to where it’s needed.
Top challenges to moving to AI-first data architecture
Today, only 25% of companies have a modern, AI-first data strategy in place, one that lets them scale AI projects across multiple lines, according to a global Omida survey of more than 400 enterprises released in May. But this is up from just 7% last year, said Omdia’s Shimmin.
“That’s a huge jump forward in just one year,” he said. “But, for most enterprises, they’re not yet at a level of maturity where a data scientist can do what a data scientist wants to do, which is build models. Instead, they’re spending the majority of their time every day trying to get the right data.”
The biggest obstacle to modernizing a company’s data architecture is the lack of expertise, said Shimmin, based on recent surveys the research firm has conducted, but it depends on the industry. For manufacturers, for example, the biggest obstacle to modernizing data architecture is the cost, he says.
“Talk to any enterprise in any market,” he says. “It’s hard to get money to invest in something that doesn’t seem to have a direct impact on the bottom line.”
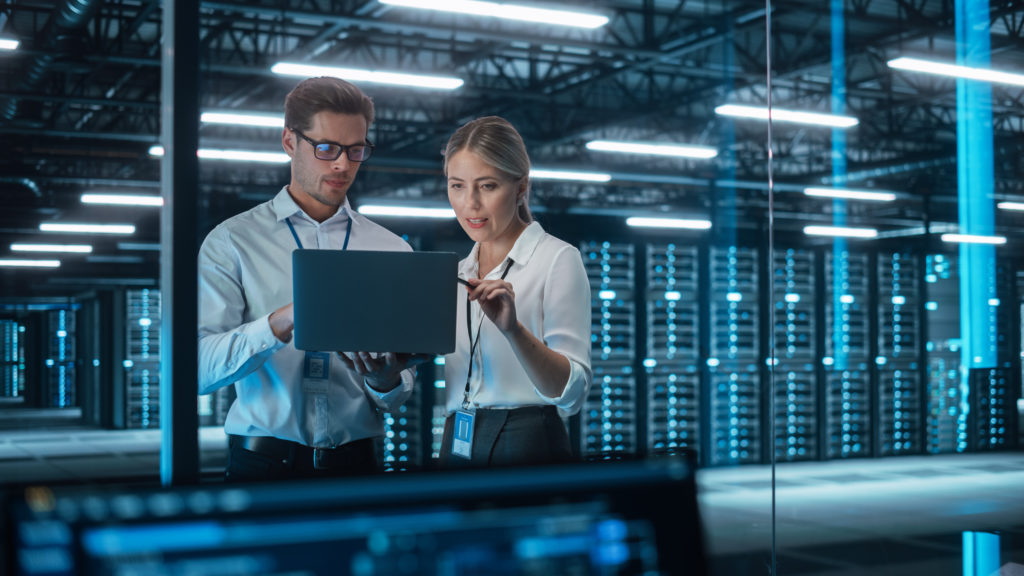
Putting AI first can also create new compliance and security challenges for companies. With more data being collected, more data needs to be secured. And more data needs to be screened for privacy and other compliance requirements. Plus, the process of combining data from two compliance sources can result in data that’s no longer in compliance, said Omdia’s Shimmin.
“That’s the challenge once the data starts moving more freely,” he said. “You might run into situations where you’re out of compliance and not know it.” To deal with this situation, enterprises should look at data management tools that can manage metadata, he said.
AI systems also create new kinds of compliance concerns. A data set might satisfy privacy requirements, but might not be representative. That will cause the AI trained on it to have a bias.
Change management issues, such as the need to break up business silos, have also been a struggle for some companies. That’s where some of the newer data strategies, like data fabrics, can make a big difference, he said.
“The data fabric is an API-driven data layer that can pull from your big database queries — but also from spreadsheets sitting on someone’s desk,” he says. “And it can turn that data into a queryable asset that can be accessed by anyone at the company. I think we’ll start to see more value coming from that.”